Understanding RDP VAE A Comprehensive Dive into the Realm of Reinforcement Learning and Variational Autoencoders
In the ever-evolving landscape of artificial intelligence, researchers continually strive to combine distinct methodologies to solve complex problems. One such fusion is the RDP VAE, an innovative approach that marries Reinforcement Learning (RL) with Variational Autoencoders (VAEs). This article aims to shed light on this powerful combination and its potential implications.
Reinforcement Learning, a subfield of machine learning, focuses on training agents to make a series of decisions in an environment to maximize a cumulative reward. It mimics the learning process of humans and animals, where actions are taken based on the feedback received from the surroundings. On the other hand, Variational Autoencoders are generative models that learn to encode input data into a latent space and then decode it back, effectively learning a probabilistic distribution over the data.
The RDP VAE concept combines these two paradigms in a novel way. The acronym 'RDP' stands for Reinforcement Distribution Policy, which signifies the integration of RL's decision-making prowess with VAE's ability to model complex distributions. The 'VAE' part refers to the use of Variational Autoencoders as a tool to learn and represent the state space in RL problems.
The core idea behind RDP VAE lies in leveraging VAEs to learn a compact and meaningful representation of the environment states. This compressed representation can then be used by the RL agent to make more informed decisions This compressed representation can then be used by the RL agent to make more informed decisions
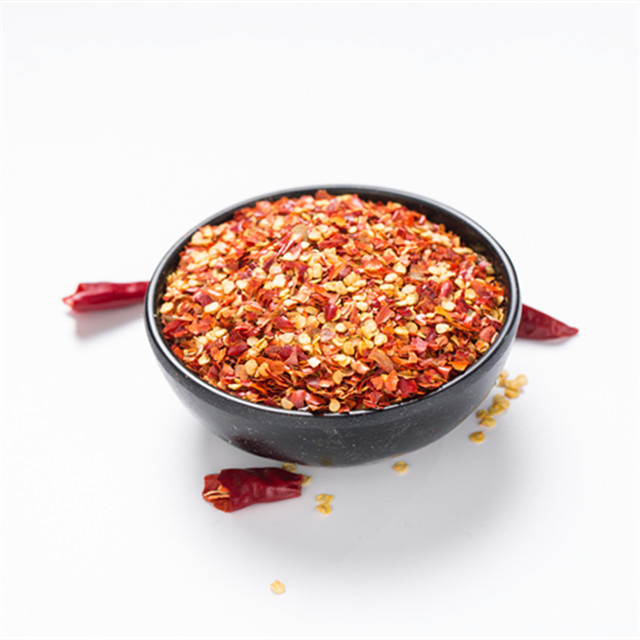
This compressed representation can then be used by the RL agent to make more informed decisions This compressed representation can then be used by the RL agent to make more informed decisions
rdp vae. By doing so, RDP VAE not only reduces the dimensionality of the problem but also enhances the agent's understanding of the environment dynamics.
One of the key advantages of RDP VAE is its ability to handle high-dimensional and complex state spaces, which can be challenging for traditional RL algorithms. It also allows for better generalization across different environments due to the learned latent representation. Moreover, the probabilistic nature of VAEs enables the model to capture uncertainty in the environment, leading to more robust decision-making.
However, implementing RDP VAE requires careful consideration of several factors, including the choice of loss functions, the architecture of the autoencoder, and the optimization strategy. Balancing exploration and exploitation in the RL component while maintaining the integrity of the VAE's learned distribution is another crucial challenge.
In conclusion, RDP VAE represents a promising step forward in the intersection of RL and VAEs. It opens up new avenues for solving complex sequential decision-making problems in domains like robotics, game AI, and even autonomous systems. As research progresses, we can expect further refinements and advancements in this field, ultimately enhancing our ability to build intelligent machines that learn and adapt in real-world scenarios.