Exploring the Potentials of RDP and VAE A Fusion for Enhanced Data Representation
In the realm of artificial intelligence and machine learning, two powerful techniques, RDP (Randomized Dimensionality Projection) and Variational Autoencoders (VAEs), have emerged as cornerstones in data analysis and generative models. These innovations, initially developed separately, have recently sparked interest in their potential fusion, offering a new dimension to the way we understand and manipulate complex data.
RDP, a statistical technique, operates by randomly projecting high-dimensional data into lower dimensions, preserving key features while discarding noise. Its appeal lies in its simplicity and ability to reduce computational complexity, making it an attractive option for large-scale datasets. However, RDP's strength is also its limitation; it may lose some fine-grained information in the projection process.
On the other hand, VAEs, a type of deep learning model, excel at learning latent representations of data. They introduce a probabilistic framework, allowing them to not only compress but also generate new, realistic samples from the learned distribution. VAEs are particularly adept at capturing the underlying structure of data, enabling them to reconstruct inputs with remarkable accuracy.
The intriguing prospect arises when these two methodologies come together. Integrating RDP into VAEs, or RDP-VAE, could potentially enhance the model's ability to capture both global and local patterns in data. By leveraging RDP's dimensionality reduction, VAEs can focus on more meaningful attributes, enhancing their learning efficiency. Moreover, the randomized nature of RDP could introduce regularization, preventing overfitting and improving generalization Moreover, the randomized nature of RDP could introduce regularization, preventing overfitting and improving generalization
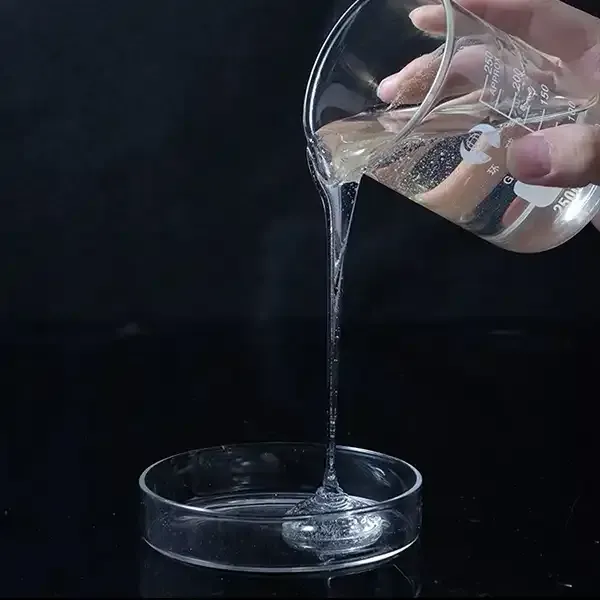
Moreover, the randomized nature of RDP could introduce regularization, preventing overfitting and improving generalization Moreover, the randomized nature of RDP could introduce regularization, preventing overfitting and improving generalization
rdp vae.
This fusion could lead to several practical applications. In image and video processing, RDP-VAEs might produce more efficient and compressed representations without sacrificing visual fidelity. In healthcare, where data is often high-dimensional, RDP-VAEs could aid in disease diagnosis by extracting essential features while preserving diagnostic relevance.
However, challenges do exist in combining these techniques. Ensuring that the randomness introduced by RDP does not disrupt the learning process and maintaining interpretability in the latent space are key areas of research. Nevertheless, the potential benefits make this combination an exciting frontier for future exploration.
In conclusion, the marriage of RDP and VAE holds promise for revolutionizing data analysis and generation. As researchers continue to delve into this fusion, we can anticipate more sophisticated tools for understanding and manipulating complex data, ultimately paving the way for innovative solutions in various industries. The synergy between RDP's dimensionality reduction and VAE's latent representation learning is a testament to the power of combining seemingly disparate techniques in the pursuit of advanced AI capabilities.